How AI-Guided R&D Is Closing the Loop on Additive Manufacturing Process Optimization


Process Optimization in Additive Manufacturing
In materials R&D, success doesn’t stop when a novel material is synthesized. In order to bring a material to life in real-world applications, Materials Development must work closely with Process Development, from the preparation and manufacturing stages, all the way to how the material performs in its end use.
A great example of this interdependency comes from additive manufacturing (AM). Here, the relationship between material and process is tightly linked: the material must be adapted to the printing process, and the process itself must be carefully tuned to the unique characteristics of each material.
Take inkjet-based AM technologies. Developing novel ink formulations is a tricky balancing act: you need to consider stability, jetting behavior, and final product performance all at once. Even after months of developing promising formulations, many fail during printhead jetting tests, plagued by issues like bleeding, short open time, or clogging.
The challenge is just as formidable in other AM domains, like powder bed or filament-based technologies, where precise alignment between material and process is crucial to meet the customer’s performance requirements.
Why Traditional Process Optimization Struggles
Traditionally, process optimization relies on Design of Experiments (DoE): systematically testing process parameters to identify which factors have the biggest impact on material behavior, and whether that impact is positive or negative.
Even when guided by expert knowledge, however, this approach faces a major obstacle — the problem space is highly multidimensional and interdependent. That makes it extremely difficult to fully map or understand, let alone pinpoint the optimal solution.
Where AI and Machine Learning Make the Difference
This is precisely where Materials Zone’s AI and machine learning (ML) capabilities excel. The platform is specifically designed to address the complexities of materials development and process optimization, challenges commonly characterized by large, multidimensional datasets spanning formulations, processing parameters, and performance results.
By analyzing both experimental and historical data within the platform, MaterialsZone’s ML algorithms uncover hidden patterns, correlations, and dependencies that are often missed through manual analysis. These insights power predictive models that forecast experimental outcomes in real time, even across highly complex material systems.
Leveraging these models, MaterialsZone’s AI guides users to map the entire formulation or process space more efficiently, helping researchers and engineers identify optimal experimental directions with fewer iterations. This data-driven approach translates into faster time-to-market, lower R&D costs, and smarter resource allocation, enabling innovation at scale in industries that depend on advanced materials.
How MaterialsZone’s AI/ML Tools Reduce Iterations and Speed Up Results
Let’s dive into three concrete ways MaterialsZone’s AI/ML tools can dramatically accelerate process optimization and help teams close the loop between material and process.
Predictive Screening
Every process engineering project generates a wealth of experimental data and insights. These findings are typically gathered, analyzed, and distilled into project reports, strengthening the researcher’s domain knowledge and intuition.
However, much of this valuable experimental history remains siloed and underutilized, rarely leveraged to accelerate parallel or future projects.
With MaterialsZone’s machine learning capabilities, historical experimental data is transformed into powerful, predictive models. These models learn how input parameters (such as material composition, viscosity, frequency, temperature, or feed rate) impact critical outcomes like drop radius, void volume, or flexural strength.
Armed with these insights, researchers using MaterialsZone’s platform can simulate the results of untested experiments virtually, before ever stepping into the lab. Rather than relying solely on trial-and-error, they can leverage past data to prioritize the most promising experiments, focusing time and resources on what matters most.

As with any machine learning system, the more data the platform ingests, the stronger the predictive models become. Each new experiment, whether successful, failed, or inconclusive, further refines the models’ accuracy. This creates a self-reinforcing cycle of improvement, driving deeper insights over time.
The result? Researchers can conduct fewer but more targeted, valuable experiments, dramatically enhancing the efficiency, speed, and success of their process optimization efforts with MaterialsZone.
Multidimensional Parameter Tuning
Many process optimization challenges aren’t just about improving a single outcome, but rather they require balancing multiple, often competing objectives simultaneously. For example, you might need to maximize AM throughput while minimizing defect rates, or improve mechanical strength without compromising flexibility.
This is where MaterialsZone’s AI-Guided multidimensional optimization capabilities make a transformative impact.

MaterialsZone’s AI-Guided R&D leverages machine learning models not merely to analyze historical data, but to actively guide the next round of experiments. By pinpointing regions in the parameter space where the models have the greatest uncertainty or where the potential for improvement is highest, the system ensures that each new experiment delivers maximum informational value.
At the same time, multi-objective optimization tools enable researchers to explore the trade-offs between competing goals. Rather than optimizing one parameter at a time or chasing a single performance metric, MaterialsZone’s AI maps the Pareto front—the set of optimal solutions where no objective can be improved without compromising another. This gives research and engineering teams a clear, data-driven understanding of their best possible options across all critical criteria.
With these advanced tools, researchers using MaterialsZone’s platform don’t adjust parameters blindly, rather they navigate complexity intelligently. Every experiment becomes more targeted, and every insight contributes to achieving finely balanced, high-performance outcomes across multiple objectives.
Starting from Zero
One of the biggest misconceptions about AI in R&D is that it only works when you already have large datasets. But what if you’re starting a new project, exploring an unfamiliar material system, or entering a space where no historical data exists?
This is where MaterialsZone’s adaptable AI tools truly shine. Even in data-scarce environments, the platform leverages transfer learning, a technique that applies knowledge gained from related projects or similar material systems to provide an informed starting point. This allows researchers to benefit from cross-domain insights, even when their specific system lacks direct precedent.

In addition, MaterialsZone’s AI integrates seamlessly with physics-informed models and domain expertise, embedding established scientific principles into its predictive algorithms. This fusion ensures that early-stage guidance is not only data-driven, but also physically plausible and aligned with foundational knowledge.
Perhaps most critically, the platform employs active learning strategies that are especially powerful during the initial phases of experimentation. These algorithms intelligently recommend the first set of experiments likely to yield the greatest informational value, enabling researchers to build effective datasets with fewer trials. Instead of relying on intuition or exhaustive design-of-experiments (DoE) campaigns, teams can quickly zero in on experiments that unlock meaningful insights about the new system.
In short, even when starting from zero, MaterialsZone’s AI ensures that researchers never start blind. It provides intelligent, strategic direction from the very first steps—accelerating learning curves, reducing waste, and paving the way for faster breakthroughs.
Accelerating Innovation and Enabling Circularity
AI-guided R&D doesn’t just accelerate process optimization, it fundamentally transforms what’s possible in the development and scaling of novel materials, particularly in demanding fields such as additive manufacturing.
By significantly reducing the time and resources needed to fine-tune material–process combinations, MaterialsZone’s AI platform empowers researchers and engineers to push the boundaries of innovation. This acceleration is especially critical as industries transition toward sustainable, circular feedstock materials, where properties can vary dynamically between batches or sources.
Unlike traditional, linear manufacturing inputs, circular materials (such as recycled powders, bio-based feedstocks, or reclaimed polymers) often introduce unpredictable fluctuations in key attributes. Successfully integrating these sustainable materials requires manufacturing processes that are highly adaptive, capable of continuously adjusting to shifting input characteristics without compromising product quality or performance.
Materials Zone’s AI and machine learning solutions make this adaptability possible. By closing the loop between material inputs, process parameters, and performance outcomes, the platform equips manufacturers to not only innovate faster but also build resilient, flexible production systems capable of embracing the inherent complexity of circularity.
In short, the future of materials innovation and the shift toward a more sustainable, circular economy will be shaped by those who can leverage AI to navigate complexity, accelerate discovery, and transform variability into opportunity. With MaterialsZone, that future is within reach!
Quantified Impact and Strategic Value
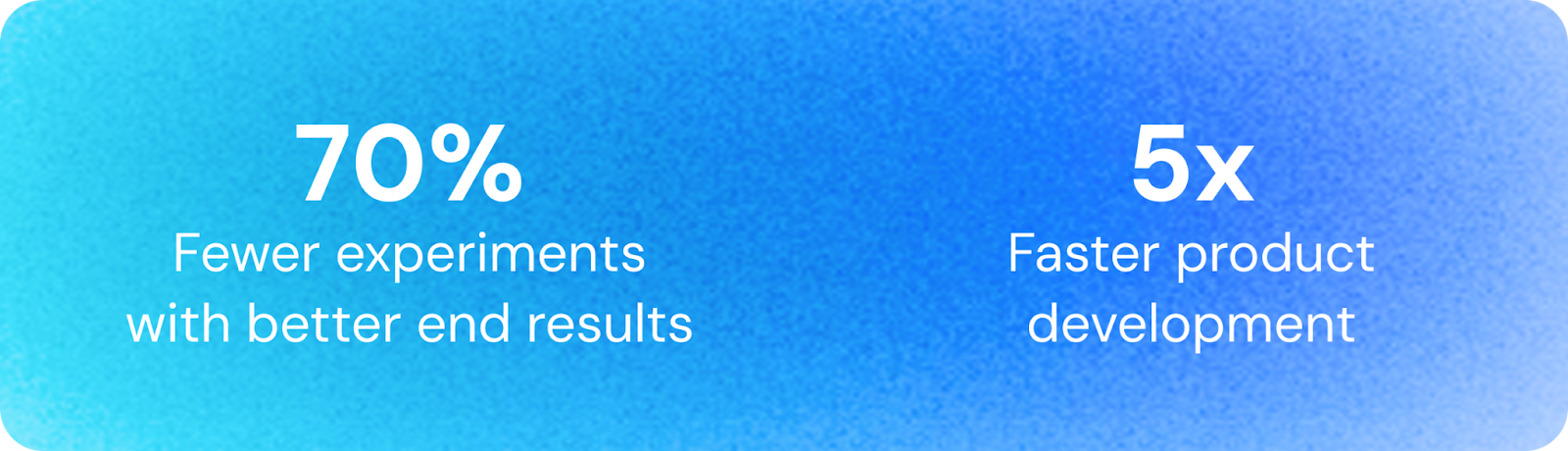
MaterialsZone’s AI-Guided Process Optimization leads organizations to:
- 50-70% fewer optimization trials
- 5x Shorter material validation pipelines
- Significant reduction in R&D costs