Energy Storage Materials
Your handheld device runs on one of billions of batteries that are produced for individual, institutional, and commercial use yearly. Battery is an assembly of multiple energy storage materials in a designed stack to create an energy storage device.
Remotes, phones, toys, watches, smoke detectors, earphones and many other everyday devices are powered with batteries. The ability to hold power has enabled us to embrace mobile devices and in essence, batteries and energy storage materials have revolutionized our lives.
Globally, the battery market size was valued at ~$110B in 2019 and is expected to grow at a compound annual growth rate (CAGR) of 14.1% from 2020 to 2027. The market growth is attributed to the high demand for automotive applications. Scooters, cars, and buses have charging stations at key positions in most advanced countries (and the numbers are growing), meaning the concept of an electric vehicle is not a far fetched for many.
Challenges in developing and producing energy storage materials and devices
Unlike developing a new plastics formulation, developing a new battery technology or to invent a new and novel energy storage material could take years of R&D work.
Without going into details about current collectors, wiring, etc., the three basic building blocks of an energy storage device are (see fig. 1):
1. Anode.
2. Cathode.
3. Electrolyte.

Each building block (or a component) has tens of variables affecting its performance. These variables can be chemistry-, composition- or process-related. Overall, this makes battery and energy storage materials development a highly dimensional problem that a human mind can’t handle at a once.
MaterialsZone is working with companies developing new battery technologies, hence, we noticed three main challenges:
• Being a highly dimensional problem, it makes it difficult for the researcher to plot data and analyze all in once. This prevents the researcher / scientist to see the trees from the forest. And hence, results in trial-and-error experimentation methodology instead of data-driven decisions.
• Long experiment cycles to capture new data points. A typical battery cycling experiment takes 2-3 months long in the cycling instrument. Once the experiment is finished, several performance indicators for that one battery device are obtained. Meaning a single data point from a device being cycled for 2-3 months! In other words, it takes a long time to obtain experimentational results.
• Raw data outputs such as current and voltage vs time, shown in figure 2, require processing and calculations to determine main performance metrics. Additional manual or semi-automatic calculations are needed to calculate, plot and analyze the results obtained from a cycling instrument or a typical potentiostat.
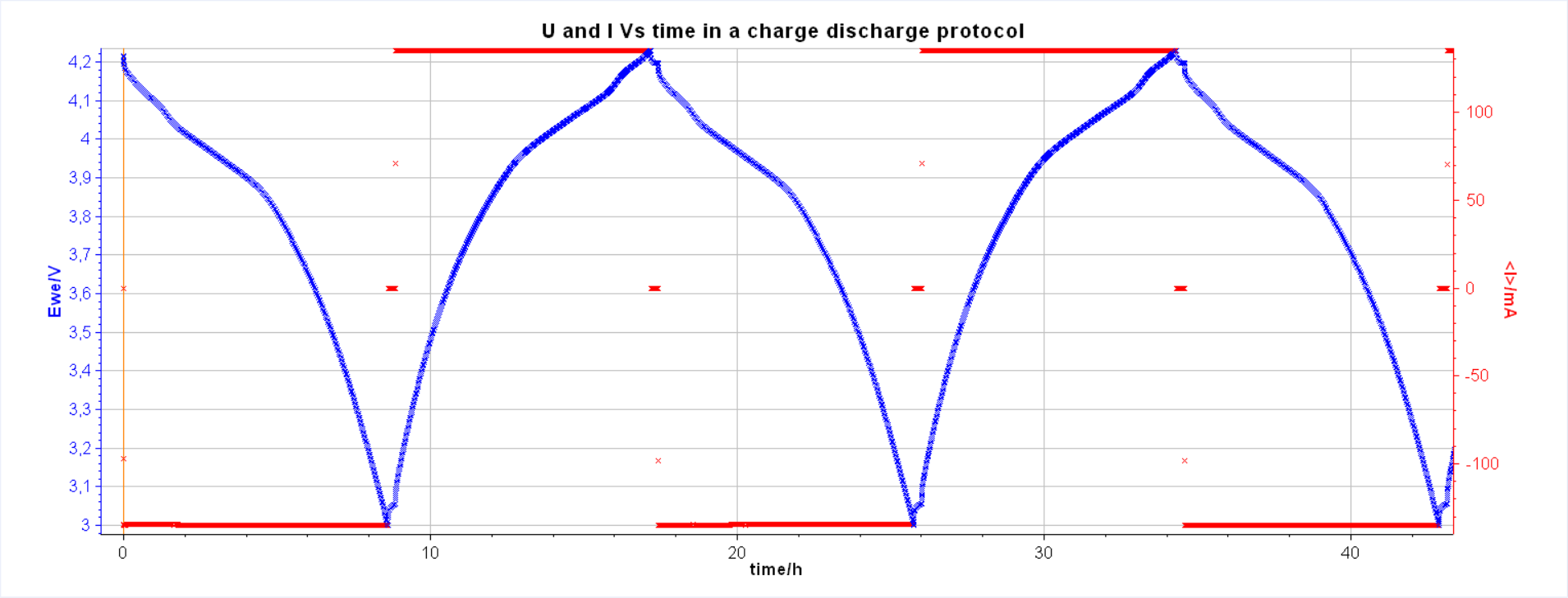
Materials Informatics Platform (MIP) as a solution for battery and energy storage materials development
Overcoming the above mentioned challenges requires an organizational materials informatics platform (MIP) that automatically harvests, parses, digitizes, stores, normalizes and harmonizes the materials-related data across the organization. Once the data is harmonized and available in the cloud-based platform, sharing, commenting, collaborating, and getting AI-based visualizations and insights across the organizational teams is as far as a click of a button.
For the use-cases in the energy storage segment, MaterialsZone has developed automations for data harvesting and normalization from characterization equipment to end up with AI/ML ready data in a cloud platform. This went through doing calculations to determine the performance indicators of the battery devices and storing the results in an AI/ML data structure for further use and manipulation. This saved the researchers / scientists redundant work of manual data extraction, calculations, and storing the results.
The AI/ML ready data already obtained with zero effort allowed the researchers, within several clicks, to plot a multi-dimensional map of correlations (figure 3) to visualize their data in a wider view without the need to plot tens of plots manually and get lost on the way.
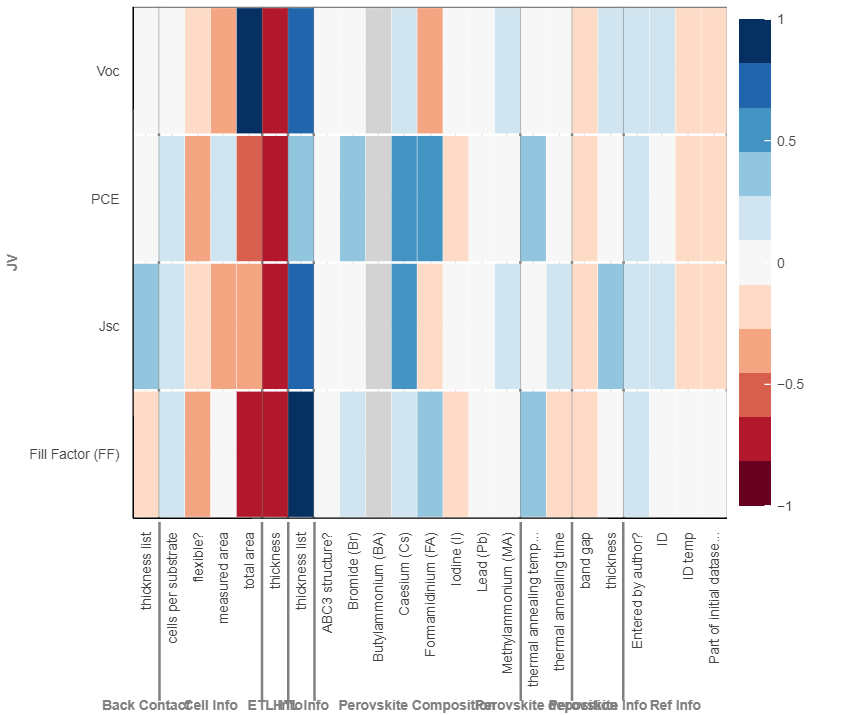
When it came to predicting the performance of a hypothetical device, one of our clients had 40-50 data points in their database / account, and they were able to make predictions to any of the descriptors they were calculating / monitoring routinely. Such predictions saved the company a lot of R&D efforts, and mainly a lot of time (each experiment takes 2-3 months to complete), and hence they focused on experiments with high probability to meet the target.