Materials Data Management
Common Challenges
• Lack of organizational memory and knowledge - The data and knowledge are distributed across the organization and its employees.
• Difficult to apply AI/ML - Since the data is spread, you need to invest a lot of effort to gather it for the purpose of conducting comprehensive data analysis.
• Not all the data is recorded - this results in waste of precious resources used to obtain the data, lack of predictability of the forecasting models and knowledge loss that may result in unnecessary repetition.
• Employee turnover results in reduction of organizational knowledge.
The value proposition of MaterialsZone
• One platform, all the data, all the insights, all the stakeholders (R&D, scale-up, manufacturing QC, supply chain alternatives selection).
• Easily applied AI/ML visualizations, insights and predictability.
• No loss of knowledge.
• Rapid accumulation of knowledge and predictability.
• Easy application of AI/ML visualizations, insights and predictions.
What does it take from a Materials Informatics Platform (MIP)?
• Flexible data model that supports multiple dimensions, multiple types of data and any hierarchical nesting and association inherent in materials data.
• Automated data harvesting including calculations and ingestion directly from instruments and any type of file (spreadsheet, PDF, binary, graphical, etc.).
• Automated data harvesting loads as AI/ML ready - very easy to slice and dice without the need of data scientists or any elaborate preparations.
• Flexibility to accumulate data incrementally and continuously while the AI/ML insights and visualizations update as well.
MaterialsZone's Solution for Materials Data Management
MaterialsZone - the materials informatics platform - allows materials data management to all users across the organization to store their information and data in a secured cloud-based platform, accessible from everywhere. Once the data is in the platform, sharing it and collaborating on it becomes easy. Further, the platform facilitates standardization across the users, hence, the data available in the platform is ready to slice-and-dice, query, visualize, analyze and conduct AI-based predictions.
Materials data management is the basics for any company developing materials-based products. Figure 1 is a screenshot from the MaterialsZone platform showing the data hierarchy and structure. This data shows partial information about a perovskite solar cell device containing materials, compositions, layers info, device stack, recipes, and device performance. Such materials data management, structure and hierarchy allows immediate conduction of big data analytics and AI-based predictions.
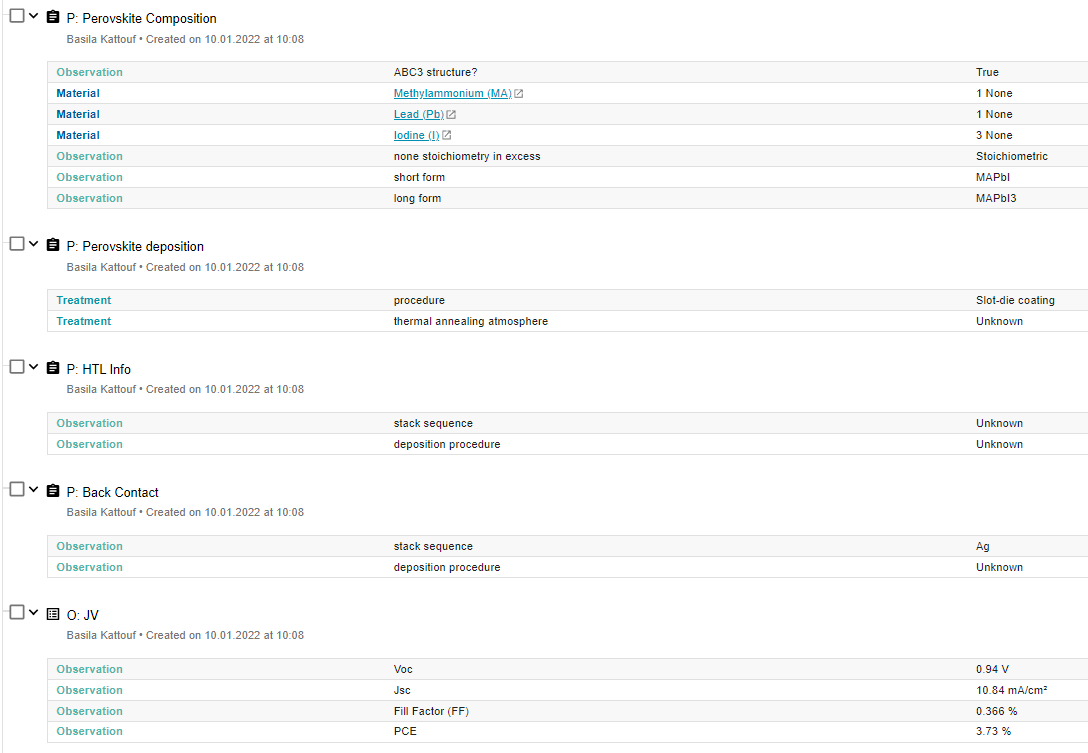
Once the data is well structured in the platform (as shown in figure 1), slicing, dicing and visualizing becomes instant. Figure 1 shows the power conversion efficiency (PCE) distribution of the devices in a given dataset containing 142 devices. Since the data were well-structured in the platform, plotting such a distribution is a matter of 3 clicks. The plot clearly shows that “C60 | BCP” as an electron transporting layer (ETL) stack sequence contributes positively to the device PCE.
